An Information Technology (IT) department with a significant ticket backlog may signal various underlying issues. For the purpose of this blog, let's define a ticket as an issue raised by a user to notify the company that their technology is not performing as expected.
Regardless of the cause, the outcome is unsatisfied customers. In fact, the problem may even compound itself as customers submit multiple tickets in the hope of a faster response, creating a larger backlog. By leveraging Minitab Statistical Software and Predictive Analytics, IT departments can identify the root cause of the issue and address it, resulting in a smaller (or no!) backlog and happier customers.
A Real-Life Example
A large IT department aimed to reduce the time required to close service tickets as the backlog grew. To achieve this, the team lead sought to identify the scenarios (i.e., variables) that had the most significant impact on ticket closure. Understanding the causes behind the speed of ticket closure could unveil the root cause of the backlog.
To tackle the issue, the department decided to examine the tickets that closed the fastest to understand the underlying reasons. By applying these insights, they could expedite the closure of other tickets. They gathered data from the past ten months of ticket resolution and entered it into Minitab Statistical Software, which included information on staffing levels (i.e., team size), the number of completed tasks, customer satisfaction surveys post-ticket resolution and project size (measured on a scale of 1-5).
The Results
They took their data and ran it through Minitab's Predictive Analytics module to clarify which variables had the most significant impact on results. Since they weren't data scientists, they utilized Automated Machine Learning, which is conveniently located within the module. This tool helped them build a predictive model within seconds and enabled them to identify the most accurate model to use, effectively identifying the key factors in ticket closure. Without the need to look at equations, the team's initial observation centered on the relative variable importance chart, shown here:
This initial data showed that the most important factor for speed of ticket resolution is team size (i.e. the staffing level). Interestingly, tasks completed ranked a distant second, while neither customer satisfaction surveys nor project size seemed to have a significant impact.
On the surface, this data made perfect sense to the team lead—the larger the team, the faster the team would resolve issues.
What is Automated Machine Learning? Minitab's Chief Marketing and Strategic Planning Officer Explains
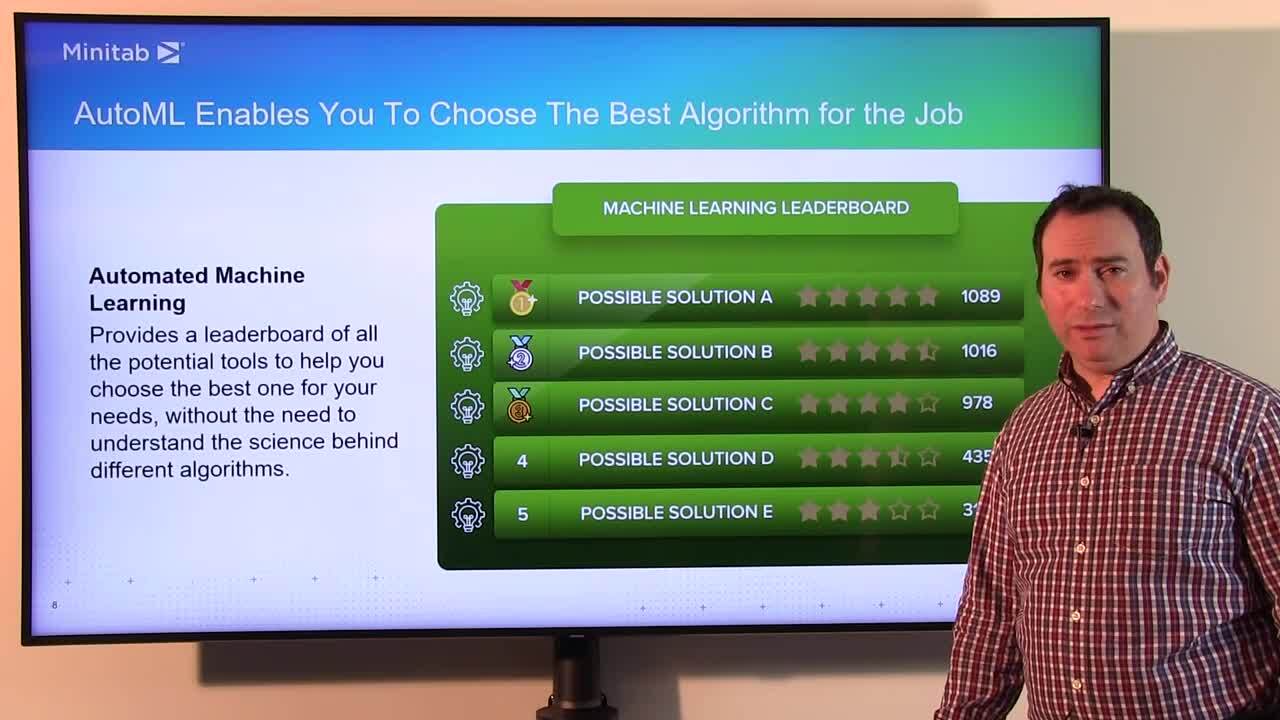
Leveraging Analytics for Deeper Insights
Armed with this information, the team now had sufficient grounds to advocate for additional staffing, but how much? The last thing they wanted to do was hire another person and gain no improvement! They needed to understand how to efficiently and effectively deploy their resources.
Fortunately, Minitab’s Automated Machine Learning tool also equipped the team with another valuable resource: the one predictor partial dependence plots. Given that the team identified team size as the most influential predictor, they could spend more time analyzing a graph of that factor. Minitab produced the following results:
We see the average results from previous tasks based on team size. The data shows us that there is a significant difference in the time it took for the team to resolve issues on a team of seven (roughly 6 days) versus a team of ten (about 2.5 days), over double, in fact.
But the data also shows us that there is no apparent difference between the time it took to resolve tickets on a team of ten versus a team of twelve. So, in this instance, it makes the most sense to dedicate a team of ten IT professionals to field tickets and problem-solve for maximum efficiency. Equally important, it demonstrates that unless a substantial investment is made in staffing levels, there's little difference between five and seven professionals, so hiring one additional team member will yield minimal impact.
Download our One-pager, 3 Common Problems Solved by Predictive Analytics, to Learn More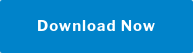
The Takeaway
This outcome is crucial because, fundamentally, it offers a roadmap for improvement and an explanation for the current problem. There's a clear solution to trim down the ticket backlog and rapidly improve customer satisfaction.
Moreover, the visualizations generated tell a compelling story to C-suite decision-makers, not just emphasizing the need to invest in staffing, but also how much staffing is necessary to rectify the issue.